KDD 2021 Honors Recipients of the SIGKDD Best Paper Awards
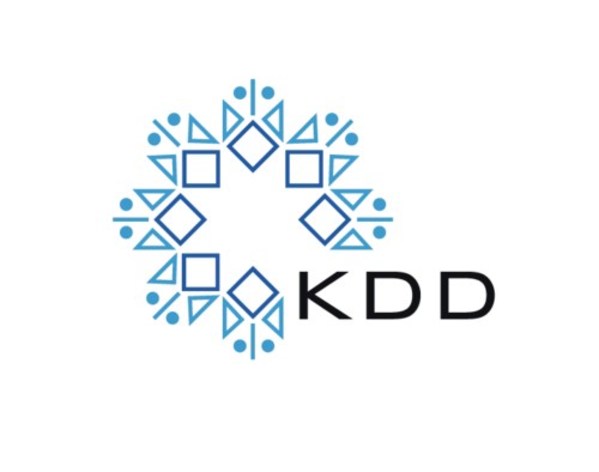
Top Data Scientists Recognized for Advanced Research and Applied Data Science in Topics Spanning COVID-19,Disaster Work Zones,and Diverse Time Range Queries
SAN DIEGO,Sept. 29,2021 --TheAssociation for Computing Machinery(ACM) Special Interest Group onKnowledge Discovery and Data Mining (SIGKDD) today announced the recipients of the SIGKDD Best Paper Awards,recognizing papers presented at the annual SIGKDD conference that advance the fundamental understanding of the field of knowledge discovery in data and data mining. Winners were selected from more than 2,200 papers initially submitted for consideration to be presented at KDD 2021,which took place Aug. 14-18. Of the 394 papers chosen for the conference,three awards were granted: Best Paper in the Research Track,Best Paper in the Applied Data Science Track,and Best Student Paper.
"Academic and industrial researchers from all over the world submitted papers to KDD 2021 to showcase the newest innovations in the field of machine learning knowledge discovery," noted Dr. Haixun Wang,chair of the SIGKDD award committee. "Those selected for recognition have pushed the frontier of machine learning especially in tackling real-world problems." The SIGKDD Best Papers of 2021 are as follows:
Research Track: "Fast and Memory-Efficient Tucker Decomposition for Answering Diverse Time Range Queries," by Jun-gi Jang and U Kang (both from Seoul National University) — Having investigated methods that analyze dense tensors to discover hidden factors,researchers showed that the Zoom-Tucker is a fast and memory-efficient Tucker decomposition method for finding hidden factors of temporal tensor data in an arbitrary time range. The paper illustrates that by elaborately decoupling the preprocessed results included in diverse time range and carefully determining the order of computations,the Zoom-Tucker method is up to 171.9x faster and requires up to 230x less space than existing methods,providing a creative solution that yielded astounding results.
Research Track,Student Paper: "Spectral Clustering of Attributed Multi-Relational Graphs," by Ylli Sadikaj (University of Vienna),Yllka Velaj (University of Vienna),Sahar Behzadi Soheil (University of Vienna),and Claudia Plant (University of Vienna) — Having investigated the challenge of graph clustering when complex data in many domains are represented as both attributed and multi-relational networks,researchers proposed SpectralMix,a joint dimensionality reduction technique for multi-relational graphs with categorical node attributes. SpectralMix integrates all information available from the attributes,the different types of relations,and the graph structure to enable a sound interpretation of the clustering results.
Applied Data Science Track: "Supporting COVID-19 Policy Response with Large-Scale Mobility-Based Modeling," by Serina Chang (Stanford University),Mandy Wilson (University of Virginia),Bryan Leroy Lewis (University of Virginia),Zakaria Mehrab (University of Virginia),Emma J. Pierson (Microsoft Research),Pang Wei Koh (Stanford University),Jaline Gerardin (Northwestern University),Beth Red Bird (Northwestern University),David Grusky (Stanford University),Madhav Marathe (University of Virginia),and Jure Lesovec (Stanford University) — The authors introduced a decision-support tool that utilizes large-scale data and epidemiological modeling to quantify the impact of changes in mobility on infection rates. The model captured the spread of COVID-19 by using a fine-grained,dynamic mobility network that encodes the hourly movements of people from neighborhoods to individual places,with more than 3 billion hourly edges. The paper describes the robust computational infrastructure required to support millions of model realizations that can simulate a wide variety of reopening plans,giving policymakers an analytical tool to assess the tradeoffs between future infections and mobility restrictions.
Applied Data Science Track,Runner Up: "Energy-Efficient 3D Vehicular Crowdsourcing for Disaster Response by Distributed Deep Reinforcement Learning," by Hao Wang (Beijing Institute of Technology),Chi (Harold) Liu (Beijing Institute of Technology),Zipeng Dai (Beijing Institute of Technology),Jian Tang (DiDi Chuxing),and Guoren Wang (Beijing Institute of Technology) — The authors presented DRL-DisasterVC(3D),a distributed deep reinforcement learning framework,to maximize the amount of collected data from unmanned vehicles in a 3-dimensional (3D) disaster work zone. The paper described a 3D convolutional neural network with multi-head-relational attention for spatial modeling and auxiliary pixel control for spatial exploration,and a novel disaster response simulator,called "DisasterSim," used to conduct extensive experiments to show that DRL-DisasterVC(3D) maximizes data collection,geographical fairness,and energy efficiency,while minimizing data dropout due to limited transmission rate.
The technical program committees for the Research Track and the Applied Data Science Track identified and nominated a highly selective group of papers for the Best Paper Awards. The nominated papers were then independently reviewed by a committee led by Chair Haixun Wang,vice president of engineering and algorithms at Instacart; Professor Wei Wang,University of California,Los Angeles; Professor Beng Chin,National University of Singapore; Professor Jiawei Han,University of Illinois at Urbana-Champaign; and Sanjay Chawla,research director of Qatar Computing Research Institute's data analytics department.
For more information on KDD 2021,please visit: https://www.kdd.org/kdd2021/.
About ACM SIGKDD:
ACM is the premier global professional organization for researchers and professionals dedicated to the advancement of the science and practice of knowledge discovery and data mining. SIGKDD is ACM's Special Interest Group on Knowledge Discovery and Data Mining. The annual KDD International Conference on Knowledge Discovery and Data Mining is the premier interdisciplinary conference for data mining,data science and analytics.
Follow KDD:
Facebook— https://www.facebook.com/SIGKDD
Twitter— https://twitter.com/kdd_news
LinkedIn—https://www.linkedin.com/groups/160888/